报告题目:Local Extreme Learning Machines (locELM): A Neural Network-Based Spectral Element-Like Method
报告时间:2021年6月7日,星期一,上午9:00-11:00(北京时间);
腾讯会议ID:797 486 725
报告人:Suchuan Dong(董素川),普渡大学
报告摘要:
Deep neural network (DNN) based PDE solvers have witnessed a dramatic growth in the past few years. Several successful ones have emerged, e.g. the deep Galerkin method (DGM) and physics-informed neural network (PINN), among others. These solvers suffer from several limitations, however, which makes them numerically less than satisfactory and computationally uncompetitive. The most prominent includes the lack of convergence with a certain rate, limited accuracy, and extremely high computational cost (very long time to train). Due to these limitations there is a general sense that these DNN-based solvers, at least in their current state, cannot compete with traditional numerical methods, except perhaps for certain problems such as high-dimensional problems.
In this talk we introduce a neural network-based method (termed locELM) for solving linear and nonlinear PDEs that exhibits a disparate computational performance from the above DNN-based PDE solvers and in a sense overcomes the above limitations. This method combines the ideas of extreme learning machines, domain decomposition and local neural networks. The field solution on each sub-domain is represented by a local feed-forward neural network, and Ck continuity is imposed on the sub-domain boundaries. Each local neural network consists of a small number of hidden layers, whose coefficients are pre-set to random values and fixed through the computation, and the training parameters consist of the output-layer coefficients. The overall neural network is trained by a linear or nonlinear least squares computation, not by the back-propagation type algorithms. We also present a block time marching scheme together with locELM for long-time simulations of time-dependent PDEs. The current method exhibits a clear sense of convergence with respect to the degrees of freedom in the neural network. Its numerical errors typically decrease exponentially as the number of training parameters or the number of training data points increases, much like the traditional spectral or spectral element type methods. We compare the current locELM method with DGM, PINN and the traditional finite element method (FEM). The numerical errors and network training time of locELM is considerably smaller, typically by orders of maginitude, than those of DGM and PINN. The computational performance of locELM is on par with the FEM in terms of accuracy and computational cost, and outperforms FEM as the problem size becomes large. A number of numerical examples will be presented to demonstrate these points.
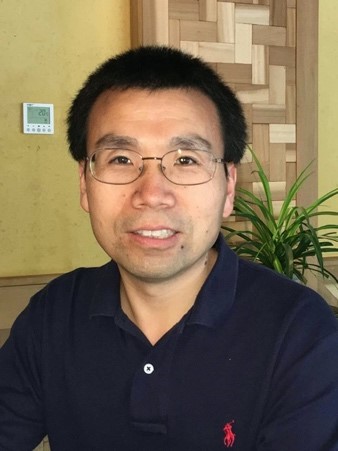
报告人简介:
Suchuan Dong is a professor in the center for computational and applied mathematics, department of mathematics at Purdue University. He received his Bachelor's degree from the National University of Defense Technology, his Master's degree from Zhejiang University, and his PhD from the State University of New York at Buffalo. He did a post-doc at Brown University. He joined Purdue University in 2006 as an Assistant Professor, and then an Associate Professor (2011) and a Full Professor (2016). His research is in the broad area of computational fluid dynamics, in particular in the development of physically consistent models, high-order numerical methods and efficient algorithms, as well as their applications. In the past few years, his research is focused on the modeling and computation of two-phase and multiphase flows, the neural network-based numerical methods and algorithms and their applications to computational mathematics and mechanics problems.